Editor's note: This year, PingWest has launched a series of discussions with China's leading pioneers and trailblazers in generative AI, amidst the rapid growth of ChatGPT-like services in China. Through these exclusive and non-exclusive talks, we aim to provide insight into the potential of Chinese AI and the thought processes of its creators.
In the first conversation, we spoke with Robin Li, the co-founder, chairman and CEO of Baidu, who unveiled and beta-tested Ernie in March, along with Geek Park, and CSDN. The interview was originally conducted in Chinese and can be read here.
On the evening of March 23, after a week of closed beta testing of Ernie Bot, Baidu’s much-awaited artificial intelligence-powered chatbot, Robin Li chatted with us for an hour.
Regarding the considerations behind the rapid invitation for closed beta testing of Ernie Bot, the comparison with ChatGPT and GPT-4, the competition in the future domestic market, and the opportunities and challenges that this technological revolution brings to humanity, he has given his answers.
Birth of Ernie Bot
"I set a deadline back then, saying that we must have the closed beta in March.”
Geek Park: Robin, as the first product in China similar to ChatGPT, there are rumors that Baidu went through a 40-day sprint to quickly deliver the product. What happened during those 40 days? Can you tell us about the process of its creation?
Robin Li: I haven't heard of the 40-day claim. Baidu has been working on artificial intelligence for over a decade. We've been working on language models for several years. From the release of Wenxin 1.0 in 2019 to now, which is almost four years, we have released Wenxin 1.0, 2.0, and 3.0. After trying ChatGPT last November 30th, we were amazed. Compared with our previous large language models, it had significant progress, especially in content generation. Since then, we have been under more pressure.
In the Chinese industry environment, including those I have met, many people asked whether Baidu will create a similar product. It's a natural direction to think of. Baidu has been focusing on language models for years, and from 2019 until now, I have spent a lot of time discussing with my team the development direction, potential applications, and how much resources we should invest in large language models. In the end, we realized that we needed to create a language model that can compete with ChatGPT as soon as possible.
Therefore, we did experience some pressure during the two months before the internal testing invitation. The team worked day and night, and we had a strong sense of crisis. When we first created it, the effect wasn't good, and we were uncertain about when we could invite internal testing. We kept discussing when we could launch it.
I set a deadline back then, saying that we must have the closed beta in March. The team wasn't confident that we could deliver it by March. I intentionally put pressure on the team to make them work faster and improve the speed of progress.
The last two months were indeed tense, but I am still satisfied. When we announced the internal testing in March, the team thought it was on March 31st. Later, I told them that I would attend the Yabuli Forum on March 17th. At that time, Ernie Bot was already gaining attention. If we didn't have internal testing by then, I wouldn't know what to say. People were interested in Ernie Bot, and I couldn't talk about anything else. Some good friends asked about it, and it's not appropriate to say nothing or reveal confidential information. After all, we are a listed company, and investors are also interested in Ernie Bot. Selectively disclosing information to some people and not to others is not acceptable. Therefore, at the end of February, I said we would invite internal testing on March 16th. It was a bit rushed at the end, but I was still satisfied with the level that Ernie Bot had achieved by March 16th.
"In such a strong market demand, it is very significant to be the first one to do it.”
PingWest: Robin, you and your team have recently emphasized that Baidu is the first company among global giants to develop a generative AI model. The tolerance of the outside world for startups and giants to develop large language models is different. What is your view on the significance of the first internal testing of large language models by giants, why is it important for Baidu, and what advantages do giants have in this regard?
Robin Li: There have been some critical voices after the internal testing of Ernie Bot, which is something I expected. I have said at least three times during the press conference that “Wenxin Yiyuan” is not perfect yet, and the main reason for inviting internal testing at this time is the strong market demand.
When ChatGPT was first released, it also faced a lot of criticism, and many people felt that it was unacceptable for someone to "talk nonsense in a serious manner". I remember that the programmer community Stack OverFlow explicitly prohibits the publication of content generated through ChatGPT in the community because the error rate of ChatGPT is too high and it is easy to mislead users. Therefore, no matter when Ernie Bot comes out, it will never be perfect. Only after it comes out can it have the opportunity to iterate and improve faster.
It is of great significance that Baidu is the first among global giants to develop a generative language model. I am very proud of this because the market demand is too strong. Countless people who were not in contact with me before or people who were far away from my industry are now asking how they can cooperate with Baidu and how they can try it out as soon as possible.
So it is very meaningful to develop a generative model early in the Chinese market. Other giants including Google, Facebook, and Amazon in the United States have not released it. I think there are two reasons. One reason is that they did not attach much importance to this matter before. The generative AI is different from the discriminative AI commonly used in search engines in the past. The algorithms, concepts, and even the standards for judging quality are different. Therefore, generative AI was not a direction that giants attached much importance to before. After ChatGPT came out, it really takes time for them to catch up with OpenAI.
When customers raise their requirements to us, we optimize and iterate in a targeted manner, and it quickly becomes very useful. For us, if the customer is not willing to pay for this, this product or technology is not valuable to us. If the customer is willing to pay for it, no matter how imperfect it is, it proves its value by itself. Therefore, I think it is very significant for whoever develops it first in such a strong market demand. When you are the second to come out as a giant, the public, or customers and the media have completely different requirements for you, so from this perspective, I also think that the first to come out is very important.
Competitiveness
"We can't control others, we just need to focus on ourselves.”
Geek Park: Comparing today's Ernie Bot with ChatGPT, which has been circulating for several months, may not be fair. Everyone is still expecting a more understandable benchmark of these two models. For example, can you define what level of ChatGPT today's Wenxin Yiyuan corresponds to in terms of technical proficiency, or which stage of ChatGPT it is comparable to? Is there a concrete benchmark that everyone can understand? Additionally, I would like to ask, although there is currently a gap between the two products, is Ernie Bot catching up in terms of computing power, data, or more innovative modeling methods?
Robin Li: After inviting the beta test of Ernie Bot, I saw various evaluations and comparisons online, all of which were comparing Wenxin Yiyu with the most advanced large language models. Not only would they compare with the GPT-3.5 version, but also with the GPT-4 version.
GPT-4 was released one day before the beta test of Wenxin Yiyu. After its release, everyone online evaluated and compared Wenxin Yiyu with GPT-4, talking about the pros and cons of each.
Many people compared the multi-modal function of Ernie Bot, which generates pictures using text, with Midjourney in any direction using the most advanced products on the market to compare with Baidu “Wenxin Yiyu”.
In fact, I think it doesn't matter whether it's fair or not. Everyone's attention and high expectations are the driving force for my continuous improvement. I also keep saying that Ernie Bot is not perfect. In fact, if you evaluate it comprehensively, Ernie Bot is indeed not as good as the best ChatGPT version now, but the gap is not very big. The so-called not very big may be a difference of one or two months. Let me tell you an internal Datapoint. About two months ago, we did an evaluation internally, comparing Ernie Bot with ChatGPT at that time. We were about 40 points behind ChatGPT at that time. We analyzed the areas where we fell behind and felt that we could solve these problems in about a month.
After about a month, we solved most of these problems and then evaluated ChatGPT and Ernie Bot again. We found that we not only did not catch up with ChatGPT, but the gap widened. So the team was very anxious at the time, feeling that we had worked for a long time and instead became worse than others.
Geek Park: Why? Is it because of data or there is other reasons?
Robin Li: ChatGPT itself is constantly upgrading, and its ability is also improving rapidly. In that month, Ernie Bot may have improved its speed, but ChatGPT may have had a major upgrade in the middle, resulting in a qualitative leap in its ability. After analyzing the gap carefully, I think that giving another month can still catch up.
According to the team's current analysis, our level is similar to ChatGPT's level in January this year. But everyone has forgotten what it was like in January, and today everyone is already used to GPT-4. GPT-4 technology is only one day away from us, and it is difficult for other major companies to come up with something to compare with it.
So I think it doesn't matter, just compare it. For me, as long as I improve fast enough and can gradually achieve things that were impossible in the past, especially when more and more users give us feedback, I still see many bright spots and directions that we have done better than ChatGPT at present. Of course, there are more directions where we are not as good as it, but I think we can make up for it over time.
Geek Park: The more people use it, even if they criticize it, the greater the possibility of catching up.
Robin Li: This is also an important reason why we urgently invited internal testing at the beginning.
Geek Park: It is also meaningful for everyone to use it while criticizing it.
Robin Li: Yes, we can't control others, we just need to focus on ourselves.
“I don't think being ‘forced’ is necessarily a bad thing”
PingWest: You mentioned that there may be elements of being "forced" in the rush to invite beta testers. So completing the beta testing is a watershed moment. In the previous stage, you may have been "forced" as the other party had already done it. But now that you are in the real environment, can you continue to be "forced"?
Robin Li: I don't think being "forced" is necessarily a bad thing. At that time, without external pressure, we might not have beta tested such a level of product so quickly. Even after beta testing, I don't think we won't be "forced" anymore.
On the contrary, we receive many more user feedbacks every day, two-thirds of which are negative. Negative feedback is actually a kind of pressure, whether it is publicly criticizing us or giving feedback through email or channels we designed. Every day, we encounter all kinds of problems, solve them, and this is the innovation process.
Naturally, our iteration speed will become faster and faster. You can call it being "forced", but I prefer to call it feedback. I have always believed that all innovation is driven by feedback. With feedback, we can continue to innovate. The more feedback we receive, the faster our innovation speed will be. Without feedback, we will be stuck in our own room every day, and there will be no way out.
PingWest: Will there be any differences in technology between Chinese and American language models in the future?
Robin Li: There will still be some differences. China has its own unique language and culture. For example, as I mentioned earlier, Ernie Bot did better than ChatGPT in some areas, such as the popular phrases in Tieba.
If you ask Ernie Bot, it will give you a correct answer with an accuracy rate of about 97% to 98%, while ChatGPT only has an accuracy rate of about 30%. I estimate that ChatGPT has less training data in this area, while Baidu has more. Another example is translating vernacular Chinese into classical Chinese or vice versa, which we are good at and obviously better than ChatGPT.
There are many other things, including when our customers require us to do targeted optimization, we can do it more finely and with higher accuracy in their field when the data comes in. Because some scenarios cannot tolerate such high error rates, we will definitely solve those problems. After a long time, there will still be many differences between these two large language models, although the basic technology is quite similar.
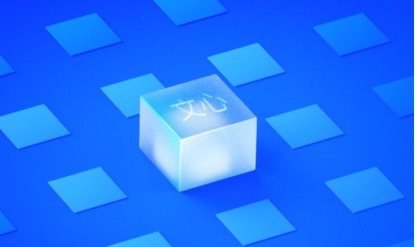
Geek Park: When observing the invitation for internal testing of Ernie Bot, especially for technical entrepreneurs, they asked whether the large language model behind Ernie Bot is completely the same as the technology route of OpenAI or has different choices. In the future, the so-called alchemy of large language models may have technological forks. When entrepreneurs choose which platform to follow for innovation, what should they pay attention to? How to choose? Will there be new variables in technology?
Robin Li: Our technology has some differences, and the most important difference is search enhancement and knowledge enhancement. Search enhancement is because it is easy to "talk nonsense seriously", and we have a very powerful search system with a high market share for more than 20 years. People have a low tolerance for mistakes in search context. When the question has relatively certain answers, we can avoid "talking nonsense seriously" by using search enhancement.
Therefore, in the demo of the news conference on March 16th, the first example used is about the author of the Three-Body Problem. I tested it many times, and the answers from ChatGPT were all wrong, while our answers were all correct. Through search enhancement, Ernie Bot first needs to understand who the author of the Three-Body Problem is and where he comes from, and what it means before asking about his birthplace. Only after getting these right can it give the correct answer.
The second difference is called knowledge enhancement, which is Baidu's contribution to the academic field of large language models. The T in ChatGPT is called Transformer, which was invented by Google, not OpenAI. ChatGPT has achieved its current status not by inventing everything itself, but by drawing on the experience of predecessors. Transformer is a new advancement for large language models. Baidu's contribution to large language models is knowledge enhancement.
In the process of doing research, we have accumulated a very large-scale knowledge graph, which should be the largest in the world, with 550 billion pairs of facts. If people's understanding of the physical world is precipitated into knowledge, expressed in one fact after another, and established a knowledge base and a knowledge graph, and then integrated it into Ernie Bot, it will make its own evolution faster because it uses some other tools, which is a resource or advantage that OpenAI as a startup company does not have.
“It is not very meaningful to disclose how many parameters there are”
Geek Park: We just talked about how Baidu has strengthened its universal large language model and made some knowledge enhancements, which is also an innovation in Baidu's large language model. I don't know if Baidu can disclose what level of parameter the universal large language model is? Is it also a training process of hundreds of billions of data?
Robin Li: It is definitely a level of hundreds of billions. This is a threshold. If it is not over a hundred billion, there will be no emergence of intelligence, which has been proven by past experiments. But it is not very meaningful to disclose how many parameters there are. After crossing the threshold of hundreds of billions, having trillion-level parameters does not necessarily have better effects than having only a hundred billion.
Before GPT-4 came out, I saw many media guessing that it had trillion-level or even ten trillion-level parameters, but the direction was wrong. large language models do not rely on increasing the scale of parameters, but on improving in other aspects. Don't be too entangled in this.
Geek Park: Do you think that enhancing certainty in choosing a technical path is a very important part for startup teams or commercial companies?
Robin Li: I think it is very important in many scenarios. In some scenarios, it may not matter if you say it wrong, but more attention is paid to creativity, tone of speech, and brilliance.
However, in situations like insurance claims, if the customer calls in and says something that needs to be paid out for an accident, and the answer is wrong, it becomes a big problem and can not be used.
In more than half of the application scenarios, the tolerance for error is very low. When there are knowledge graphs and retrieval enhancements, the more specific the industry application, the more it will show its advantages.
Technology
“Engineering is done first a lot of the time, and then slowly, the studies follow.”
PingWest: You just mentioned the relationship between theory and engineering. We also know that whether it is OpenAI doing ChatGPT or Ernie Bot. They are essentially doing things that engineers do, and they don't invest much in basic technology. Some people call this process a "large-scale experiment in violent aesthetics" because a lot of funds and computing power are invested to do it. Recently, a scientist in the AI field told me that he felt very disillusioned. Everyone participated in such experiments, like alchemy. You don't know which effort and leap caused the changes, and what is the key link that makes a large language model able to come out and run. Which month do you think is the most crucial in the past few months for this outbreak moment?
Robin Li: To put it simply, I don't know which month is the most crucial. It’s like suddenly our ability is there. But I believe that in the future, humans will definitely figure out the theoretical basis behind it. Engineering is done first a lot of the time, and then slowly, studies follow.
This is like aerodynamics and principles, which gradually emerged. We are accustomed to using theory to guide practice from childhood to adulthood. If this practice is not guided by theory, and even the current theory cannot explain it. That’s where the sense of disillusioned, unacceptable coming from. But it’s not alchemy or pseudo-science.
In fact, science itself is also developing. Why should we believe that the science we know now is the truth and everything is correct? We still need to accelerate the iteration of technology through continuous practice, innovation, and feedback. After it runs out, it is OK to slowly study the theoretical basis behind it. If it doesn't run out, in five years, people will not study in this direction.
In fact, large companies are not doing generative AI, and they have not invested too much resources in it, including the academic community. Everyone did not feel that this matter was worth so many people studying, but once it ran out, it was really amazing and attracted attention. I believe that a large number of scientists will follow up and study what the theory is behind it. Of course, after summarizing this theory, it may also be used to guide the next iteration and update of large language models, which is completely reasonable.
CSDN: I have some questions representing developers. When ChatGPT came out, it was during the NIPS conference, where 40,000 machine learning and neural network PhDs were attending. People were all amazed - it seemed to exceed our understanding of NLP or conversational abilities. Later, it was explained as the emergence of intelligent capabilities. Has this secret been revealed? Although ChatGPT did not use a lot of Chinese corpus, its understanding of Chinese seems to be poor, but it can still express Chinese very well. We chose the famous work of Chilean poet Pablo Neruda to translate into Chinese and found that it was better than the translation done by translators. What is your opinion on this breakthrough? Can you explain to technical personnel how the emergence is achieved? Why is the language gap overcome with little corpus?
Robin Li: This is indeed a surprising and exciting development. We have been working on large language models for many years, and there are actually many other companies working on large language models. When using a billion-level model to do a single task, or one or two tasks, it may be relatively narrow. Later, it became ten billion, one hundred billion, and finally the parameter scale reached 100 billion, while matching enough data for training, and finally intelligent emergence appeared, which should be said to be a process from quantitative change to qualitative change. Just three years ago, when we talked about large language models, they were models with parameter levels of billions. Today, when we talk about large language models, most people understand that these are models with parameter levels of 100 billion. This evolution and technological iteration speed actually exceeds the evolutionary speed of Moore's Law that everyone is familiar with, which is still very amazing.
Once we cross that threshold, something that we thought was unlikely has undergone a qualitative change. If we look a little bit deeper, why is there such a qualitative change? My understanding is that although it is a probability model to learn texts of various languages in the world, it is still based on the past ten characters or tokens that have appeared. The next character or token is most likely, and the simple technical principle is like this. But when the actual data volume is large enough and the algorithm is relatively correct, the understanding of the physical world by humans is gradually compressed into a model. If we understand large language models in this way, it does have the ability of intelligent emergence or analogical reasoning, which I think is really amazing.
People didn't think that many things were made and then thought about why this thing was made and what the scientific principles were inside. Because we all studied science and nature in school, our impression is that social progress and technological progress are all based on theory first, then technology and engineering are done under the guidance of theory, and then it is made into a product and pushed to the market. In fact, engineering is done first, such as people inventing airplanes first and already flying in the sky, and then people started to think about why something heavier than air can still fly in the sky, which led to the birth of aerodynamics. So large language models are a bit like this. They were made first, and then we began to study why it is like this.
CSDN: If everyone uses this hundred-billion model, can everyone gradually achieve this ability? Gradually becoming like an open-source system, where everyone knows the basic principles, but you haven't open-sourced everything. Can we also achieve this? Can other companies also achieve this?
Robin Li: Yes, this is a moving target that is constantly changing. ChatGPT itself is evolving at a very fast pace, and Wenxin Yiyu is evolving even faster. The next one that comes out, whether it's a start-up or a big company, it's definitely possible to achieve the level we have today. But we think it's already amazing today, and maybe in three months we'll find out that this thing is so bad, how can it still make mistakes. People's expectations will continue to rise, and it will be quite difficult for the next model to catch up with the previous big model. In the same market, the leading big model will definitely attract more developers to develop various applications on it, and will definitely receive more feedback from users. Once this scale effect or data flywheel starts to turn, it will be quite difficult for latercomers to catch up.
“It may be easier to find a job by studying humanities.”
CSDN: For developers, Silicon Valley has been buzzing with various GPT-based applications, bringing significant differences to programming. In the past, we focused on APIs and technology stacks. Now, we promote programming. The entire developer ecosystem and applications will undergo significant changes. What do you think about the future? What changes will occur in ToC and ToB applications beyond the model, rather than model applications?
Robin Li: I think this is a significant trend. In the future, we may not need as many programmers. Many times, the large language models can automatically generate code. However, we will need more and more prompt word engineers. The ability of the large language model itself is there. Who can use it well is the key, and it depends entirely on prompt words. If the prompt words are good, the possibility of intelligent emergence is greater, and the feedback results are more valuable. If the prompt words are not good, the output will be nonsense or incorrect conclusions. So how to write good prompt words is both a technology and an art, and I think the art component is even more significant. From a secular perspective, it seems that people who study natural sciences are better at finding jobs and have higher salaries than those who study humanities. In the future, it may be easier to find a job by studying humanities, because when writing prompt words, imagination, emotion, and expression may be more interesting and effective than those who study engineering.
CSDN: Will different large language models, such as ChatGPT or GPT-4, have different prompt words?
Robin Li: Yes, they are very different. The underlying training is independently trained, and if it is compared to a person, their temperament and character will be different. There is also a process of continuous exploration during interaction with it, and you will gradually know how to write prompt words to get better results.
CSDN: Will the data it responds to also change?
Robin Li: Yes, it will change. Recently, there has been a lot of discussion about writing idioms. You may think that it does not understand what you are saying, but after a few days, it will understand. If you keep telling it that it is wrong, it will know that it is not right, and you can redo it.
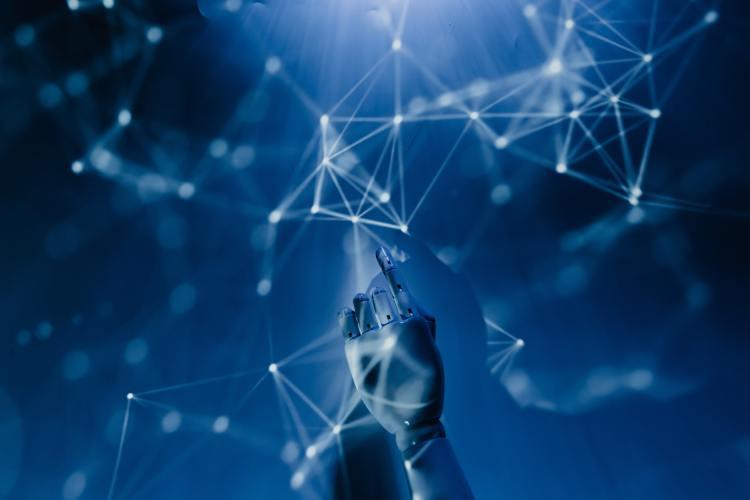
Commercialization
“Competition and commercialization will make technological advancements faster.”
PingWest: We talked earlier about OpenAI's GPT-4 not publishing papers or open-sourcing their work. Without papers, how can scientists conduct research? How can we cooperate with science and theory?
Robin Li: OpenAI is relatively commercialized now, which is not necessarily a bad thing. With sufficient funding, the pace of technological iteration will be faster. Whether to open-source or not is completely their choice. If they don't open-source, the pace of technological iteration will be faster, which can better benefit humanity and is also a good way forward. External research cannot rely solely on OpenAI's disclosures. In fact, various companies and research institutions have already started working on these machines, investing where necessary, conducting research where necessary, and trying where necessary. Therefore, I think a production-study-research model will gradually form, where everyone does their own thing, and slowly a scale of field or even discipline will emerge. I am not worried that the outside world will not understand what OpenAI is doing. The pace of iteration in this technology or direction will slow down. I actually think that competition and commercialization will make technological advancements faster.
Geek Park: Many people outside are speculating that in the future, the track for large-scale models will require continuous investment of more than tens of billions of dollars to improve technology. I'm curious, from Baidu's perspective and your perspective, is it inevitable to invest at this level? Are there any other options?
Robin Li: Investment is definitely necessary and will continue to increase. For example, the current investment level of OpenAI is over tens of billions of dollars. However, if there is competition, investment will definitely increase. Therefore, no one knows whether the future investment will be in the tens or hundreds of billions of dollars. We only know that with these investments, technological progress will be faster, and the commercialization of various industries and scenarios will also be faster. Therefore, investment is only one side of the coin, and the other side is the returns, which are indeed useful in various industries and scenarios we can think of.
Therefore, developing large-scale models not only means investment, but also means returns. These returns will become more and more evident over time. I don't know if you've read OpenAI, but they have gone from a non-profit organization to a limited organization. The threshold and profits have to exceed that of today's Apple, which is the world's first or second largest company in terms of market value. Only then will they revert to a non-profit organization. This shows that they have high expectations for business and profits, rather than just pure investment. Pure investment can not develop so quickly. There must be returns, and the fundamental reason for these returns is effectiveness and market demand, which have a positive impact on our society and civilization.
Geek Park: So, it's like practicing alchemy while generating electricity. Will Baidu quickly integrate this capability into its search engine?
Robin Li: Definitely. Currently, every department at Baidu, including search, Xiaodu, Tieba, Wenku, Wangpan, and Maps, is working overtime to research and integrate the ability to understand natural language more quickly and naturally. This integration will be so natural that you'll feel like this capability is necessary for the product. This is true for Baidu, as well as many other companies. Everyone can naturally see that they can use, integrate, and need these capabilities.
Therefore, society will evolve at a faster pace. If we look back 15 years ago, for example, before the iPhone was released, it was difficult to imagine how people lived back then. If we look back in 2023, five or ten years from now, we'll feel the same way. People in the past may have thought that life was the same for a couple of hundred years, but today, looking back 15 or 20 years, we can see that things have changed a lot. When we watch some TV shows from the 90s today, we can see that their life scenes are clearly different from today. I think this feeling will be more pronounced in the next five or ten years.
“large language models is a game changer”
PingWest: It's clear that you have a passion for technology, but you have also mentioned commercialization throughout the process. I noticed that you mentioned in the beginning that if this technology is not purchased by customers, it is actually meaningless. Some of the questions just now also focused on specific functions such as Baidu search, but we will find that the discussion on ChatGPT may have overlooked Microsoft's cloud Azure. In fact, the cloud market has undergone a very obvious change. So, how do you think big models will change the cloud market?
Robin Li: Yes, I have publicly stated that I believe the emergence of Ernie Bot or large language models is a game changer for cloud computing, and it will change the rules of the game for cloud computing. Because traditional cloud computing in the past was mainly selling computing power, mainly basic abilities such as computing speed per second and storage. However, with the evolution of technology, the applications of the real AI era will not be built on a past foundation. In addition to cloud computing mentioned earlier, there is also the development of apps on operating systems such as iOS or Android in the mobile era or various software development on Windows in the PC era. In the AI era, new applications will be developed based on large language models. Regarding the question of whether all models will eventually be unified into one model, I pushed for a period of time internally about two years ago to unify language, vision, and speech models into one model. Although everyone thought it was not right and impossible at that time, the language model will become stronger as its scale grows, and the vision model will become stronger as its scale grows.
Future applications will be developed based on these models. The development of things like search or Tieba is based on the large language models we have developed. This is different from a startup company directly using a certain cloud computing service. At that time, it was indeed computing power, even down to using several CPUs or GPUs. However, in the future, we no longer need to worry about this level. For example, I learned assembly language when I was young, then learned C language, and now everyone is writing code in Python, and the convenience level is completely different. If you can write code in Python, who will still learn assembly language? It's that simple. So, for Baidu, my theory is a four-layer architecture: chip layer, framework layer, model layer, and various applications on top. Early people said they had chips and wanted to develop various applications based on this kind of chip. Later, we said that Baidu's PaddlePaddle, the framework of the AI era, has the first market share in China, and in the United States, it is Pytorch and TensorFlow. Before 2023, developers will rely on frameworks to develop AI applications. But after the emergence of large language models, the framework will become a relatively low-level thing, and in the future, various applications will be developed based on models. What framework is used below is actually not that important.
However, for companies like Baidu, it is still important what framework and chips we use when providing basic models. In a sense, each layer strengthens each other through feedback and continuously improves its efficiency. So, it is called end-to-end optimization internally. We have Kunlun at the chip layer, PaddlePaddle at the framework layer, and Wenxin Yiyu at the large language model layer. Of course, this brute force aesthetics, as mentioned just now, is very computationally intensive. So, with the same $1 billion worth of chips, how can we be more efficient than others? How can we calculate faster? We need the PaddlePaddle framework to cooperate. The model also needs to know what abilities these chips have and how to fully utilize them, or how Kunlun chips can modify their design to be more suitable for PaddlePaddle and Wenxin Yiyu models.
After end-to-end optimization, our efficiency will be higher than any other large language model. So, over time, commercial competition will eventually compete for efficiency. If your efficiency is higher than others, you win. If your efficiency is lower than others, no matter how much money you invest, it will eventually fail. Countless cases have proven this.
PingWest: It's actually a three-tier architecture, and applications are only at the last layer. At present, it's difficult to say where GPT-4 can be widely applied in large industries. Writing papers or providing psychological counselling may not lead to large-scale industrial applications either. Based on China's industrial environment and structure, can we take a detour or change lanes instead and overtake competitors?
Robin Li: I do think that there could be an additional intermediate layer, known as the industry macro-model. In addition to these basic models, a specific industry, such as the energy industry, could have an industry macro-model which is a visible entrepreneurial opportunity for the future. Some industries may be relatively slow to react, and their clients may not feel the urgency to adopt new technologies. At this point, if you train an industry macro-model based on the commonalities of this industry, you can gradually acquire industry clients and let them develop their own applications based on this industry macro-model.
Ecology
"The biggest opportunity for startup companies lies in applications.”
PingWest: So you mean that for universal large language models, it's better for startups not to get into it, because it takes time and money. Instead, it's better to give it to some big platforms, and let them derive industry model applications based on this, which is a better ecosystem.
Robin Li: That's how it looks like right now. If startups do large basic models, they have no advantage. This is very different from the OpenAI era. After it was established in 2015, it slowly figured out a way in the direction that others didn't look at or didn't think highly of, and finally gathered a group of developers with Microsoft's support, which can have today's achievements. But now, when all the big companies are desperately investing resources, as a startup, if I want to make a large basic model and want all developers to develop applications based on my model, there is no reason for it. You are not the first one to do it, and there is already a market for it. You don't have an advantage in data, computing power, or ecology. For startups, it's better to do something new, something that others don't look good on, and the success rate will be higher, and the social significance and commercial value will be greater.
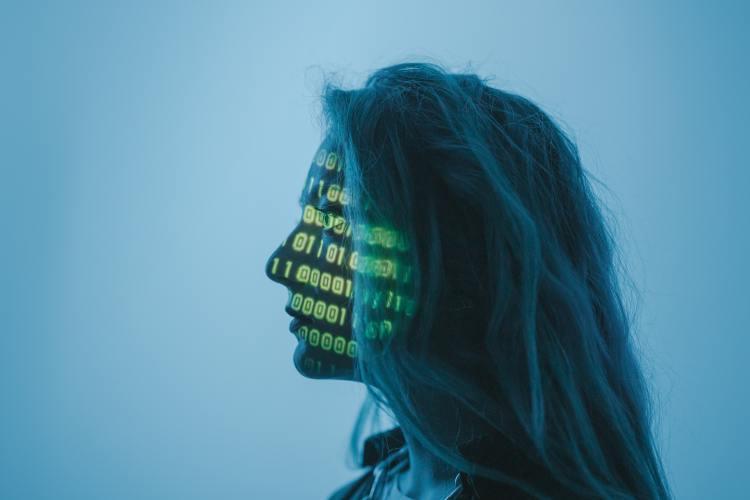
CSDN: I have a question. Everyone compares the appearance of ChatGPT to the arrival of iPhone in the mobile era, where there was a competition between open, open-source and closed-source. iOS is closed-source, while Android is open-source. In the end, open-source won a great victory for its ecosystem. Therefore, does the open-source large language model, including Meta's LLaMA, have a market opportunity?
Secondly, there are two methods for industry-wide models: one is to refine them on Baidu Wenxin Yiyuan, and the other is to practice my vertical large language model on the open-source large language model. Which one is better? Will there be such an ecosystem for open-source large language models?
Robin Li: I think it is possible, but ultimately it is a natural selection of the market. For a developer, the most important factors in choosing between a closed-source and an open-source large language model are which one has better performance and which one is cheaper. Open-source has a very clear advantage in price, and it is basically possible to use these things without paying any money. If closed-source is still viable, it must be better than open-source to survive. Therefore, when you pursue better performance, you will choose a closed-source model. However, this is a static observation or discussion. Dynamically, with the passage of time, which of the two technical routes of open-source and closed-source will run faster, have more momentum, and have better sustainability, I think this is an open question with both positive and negative examples. For developers, they can only choose a model with better performance or higher cost-effectiveness for development. We can only wait and see for the competition between these two routes.
Geek Park: One last question. In the entrepreneur community, everyone specifically asks me to ask Robin for their advice. We previously talked about the Mobile Native in the mobile era. Now, what is AI Native? Has Robin thought about it or can he share his thoughts? For entrepreneurs, should they quickly develop ToC products today, or think more seriously about how to change business logic in some vertical areas and advise entrepreneurs on how to act?
Robin Li: Today, large language models are in the very early stages of industry development. No matter what kind of observation, mine or others, changes may occur. In my opinion, the most obvious feature of AI Native is the prompt words I mentioned earlier. In the past, we did not think that there were so many requirements for interacting with computers. Today or in the future, how to write prompt words to promote the capabilities of large language models is a very interesting industry, and I also believe that this will be the easiest place for new job opportunities to emerge. I even have a bold guess that I think half of humanity's work will be related to this in 10 years, that is, writing prompt words. In addition to the major changes in prompt words, from the perspective of entrepreneurship, I believe that the opportunities will be huge, and this opportunity may be ten times that of mobile internet. The main opportunity will definitely be various applications developed based on large language models, whether it is ToC or ToB, charging or advertising models. I think there will be both. The opportunities in each direction are so great that as an individual entrepreneur, you don't need to worry about whether the market is large enough, and there is no ceiling, so a startup company doesn't have to worry about it either.
PingWest: Just take action.
Robin Li: Thank you. I had a very enjoyable chat.
Interviewers: Thomas Luo, Zhaoyang Wang